Filter articles
标签
产品
Loading...
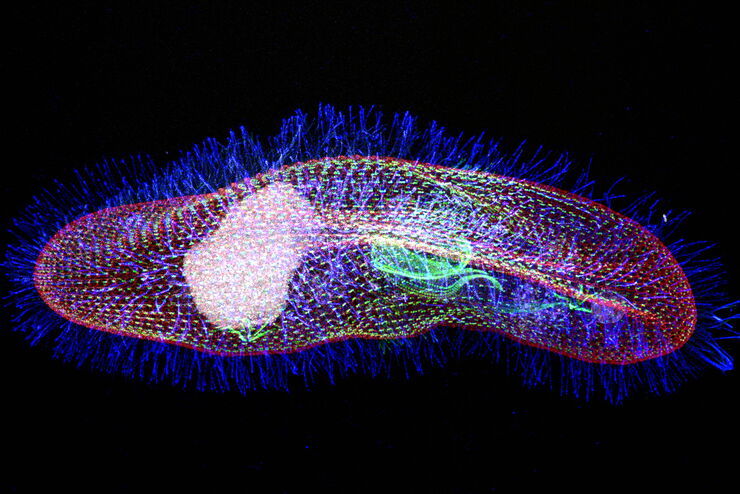
复杂3D数据集——人工智能赋能的空间数据分析
本期MicaCam为您提供切实的建议,教您从显微镜图像中提取可发表级别的分析结果。本期的特邀嘉宾来自徕卡显微系统的Luciano Lucas,他将为大家展示如何使用MICA的AI赋能软件进行图像分析。他将深度分析两张MICA的3D成像,探究不同可见生物元素之间的空间关系。本期的最后将会介绍如何创作高保真视频动画以及其他可用于发表文章的结果。
Loading...
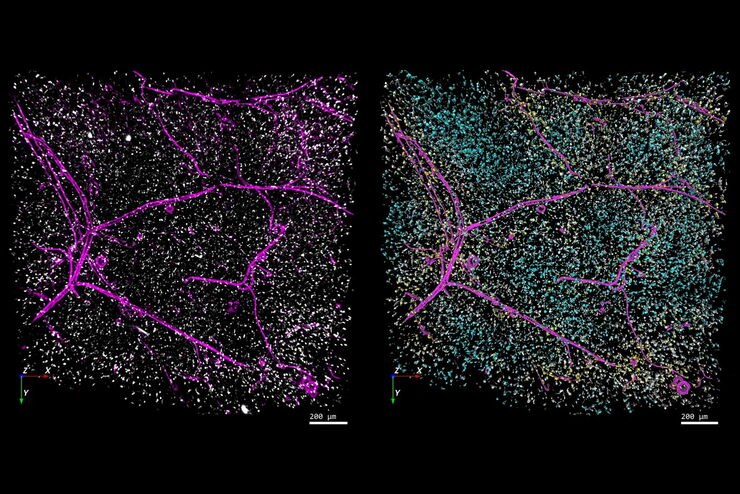
精确分析宽视野荧光图像
利用荧光显微镜的特异性,即便是使用厚样品和大尺寸样品,研究人员也能够快速轻松地准确观察和分析生物学过程和结构。然而,离焦荧光会提高背景荧光,降低对比度,影响图像的精确分割。THUNDER 与Aivia 的组合可以有效解决这一问题。前者可以消除图像模糊,后者会使用人工智能技术自动分析宽视野图像,提高操作速度和精确性。下面,我们来详细了解下这一协作方法。
Loading...
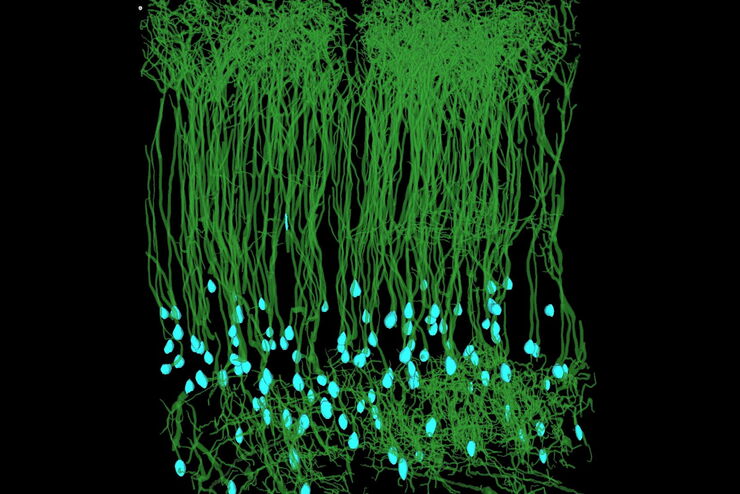
自动化加速神经元图像分析
复杂神经投射的检测能力主要取决于大规模神经元网络的精确重建。神经科学研究中的大多数数据析取方法都非常耗时和易错,进而导致进度延误和错误。在本次研讨会中,Aivia将演示如何利用自动化技术提升图像分析工作流的效率
Loading...
![[Translate to chinese:] Dynamic Signal Enhancement powered by Aivia: Truly simultaneous multicolor imaging of live cells (U2OS) in 3D [Translate to chinese:] Dynamic Signal Enhancement powered by Aivia: Truly simultaneous multicolor imaging of live cells (U2OS) in 3D](/fileadmin/_processed_/b/1/csm_How_Artificial_Intelligence_Enhances_Confocal_Imaging_teaser_bba50f917e.jpg)
人工智能如何增强共聚焦成像
在本文中,我们将展示人工智能(AI)如何增强您的成像实验。即,由 Aivia 提供支持的动态信号增强如何在捕捉活细胞样本的时间动态的同时提高图像质量。